IBM Watsonx.ai
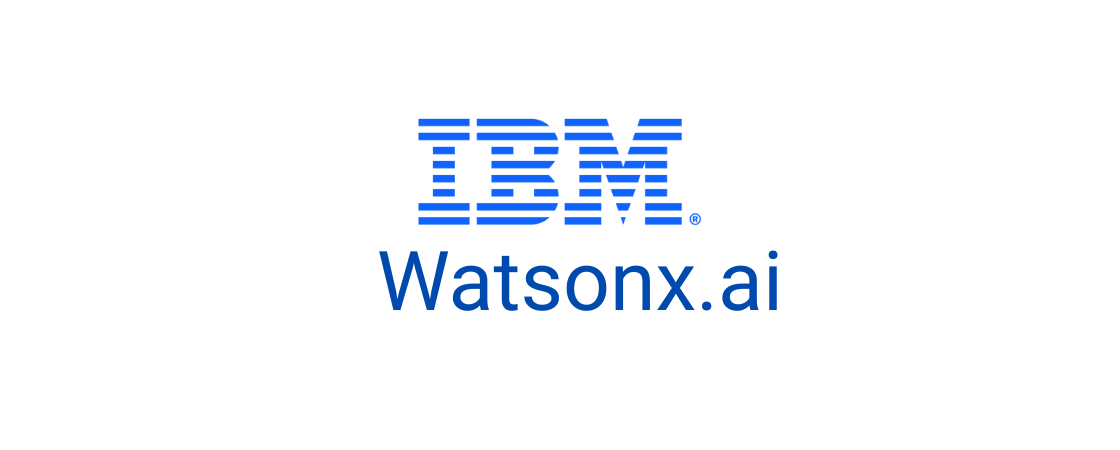
Build, Train, Tune, and Deploy foundation models, machine learning models, and custom AI.
About IBM Watsonx.ai
IBM watsonx.ai is a powerful AI development studio designed for building, training, tuning, and deploying foundation models, machine learning models, and custom AI — all in one place. It's a key part of IBM's broader watsonx platform, which includes watsonx.data (for data) and watsonx.governance (for AI oversight).
Watsonx.ai gives developers and data scientists access to:
- IBM’s curated foundation models, LLMs (e.g., Granite series)
- Open-source models (like Llama 2, Falcon, Mistral)
- Tools to fine-tune, prompt, and evaluate models
- Full lifecycle support from model training to deployment
It’s built to help organizations create enterprise-ready, trustworthy AI, including generative AI apps (like chatbots, summarizers, RAG pipelines, and code assistants).
Key Capabilities
- Foundation Models (FM Studio)
- Use IBM’s Granite models (LLMs trained on enterprise-safe data)
- Access open-source models (Llama 2, Mistral, etc.)
- Hosted in a secure, scalable environment
- Includes prompt engineering tools and evaluation dashboards
- Custom Model Training & Fine-Tuning
- Fine-tune models using your own domain-specific data
- Use techniques like supervised fine-tuning (SFT) and parameter-efficient tuning (PEFT, LoRA)
- Supports RAG (retrieval-augmented generation) for knowledge-grounded answers
- Machine Learning Studio
- Train and deploy traditional ML models using:
- AutoAI
- Jupyter Notebooks (Python, R)
- Popular ML frameworks (scikit-learn, XGBoost, TensorFlow, PyTorch)
- Model Deployment & Inference
- Deploy models as RESTful APIs securely
- Integrate easily with apps or other services
- Scale via IBM Cloud, Red Hat OpenShift, or hybrid infrastructure
- Built-in Evaluation & Guardrails
- Evaluate models for:
- Bias
- Toxicity
- Hallucinations
- Explainability
- Add custom policies and filters to align with enterprise values and regulations
How It Fits in the IBM Watsonx Suite
Component | Role |
---|---|
watsonx.ai | Train, tune, and deploy foundation models |
watsonx.data | Manage and query data for AI workloads |
watsonx.governance | Govern AI use with policy, tracking, explainability |
Together, they offer end-to-end AI lifecycle management — from data to model to deployment to compliance.
Steps to Create an AI Model in IBM Watsonx.ai
Step 1: Log in to watsonx.ai
- Go to https://dataplatform.cloud.ibm.com/
- Sign in with your IBM Cloud credentials
- Launch the watsonx.ai studio
Step 2: Set Up a Project
- Create a new project workspace
- Attach a Cloud Object Storage instance
- Define the runtime environments (e.g., GPU-based for training)
Step 3: Prepare Your Data
- Upload or connect to training data via:
- Cloud Object Storage
- watsonx.data
- External databases or files
- Data formats: JSON, CSV, Parquet, etc.
- Clean, label, and structure your data as needed (e.g., for SFT or RAG)
Step 4: Choose a Foundation Model
- Navigate to Prompt Lab or Model Catalog
- Select a base model:
- IBM Granite series (for code, NLP, etc.)
- Open-source models (LLaMA 2, Mistral, etc.)
- Certain Models are pre-trained and hosted securely
Step 5: Fine-Tune the Model
Choose your tuning strategy:
- Prompt tuning: Light-touch customization via examples
- Supervised fine-tuning (SFT): Use your labeled Q&A or instruction-following data
- Parameter-efficient tuning (e.g., LoRA): Efficient for large models
Training Steps:
- Choose model + dataset
- Configure hyperparameters (epochs, batch size, learning rate)
- Launch the fine-tuning job (runs on IBM’s GPU backend)
Step 6: Evaluate the Model
- Run test prompts and assess:
- Accuracy
- Bias
- Toxicity
- Hallucinations
- Use built-in Guardrails & Evaluation Lab to automate checks
- Iterate with further tuning if needed
Step 7: Deploy the Model
- Deploy your tuned model as a RESTful API endpoint
- Auto-generates secure API tokens and OpenAPI specs
- Integrate with your apps or workflows
Step-8: Use with watsonx.data & RAG
Enhance your model with retrieval-augmented generation (RAG):
- Connect to watsonx.data for the enterprise data and the knowledge catalogs
- Add vector search for grounding responses
- Improves factuality and domain alignment
Step 9: Monitor and Govern (Optional but Recommended)
- Use watsonx.governance to:
- Track drift
- Enforce usage policies
- Enable auditing and documentation
Retrieval-Augmented Generation (RAG) Flow
Once the LLM Model is trained and deployed, you would need this type of typical workflow understanding of a Retrieval-Augmented Generation (RAG) process to facilitate Questions and Answers to the users via a Chat interface.
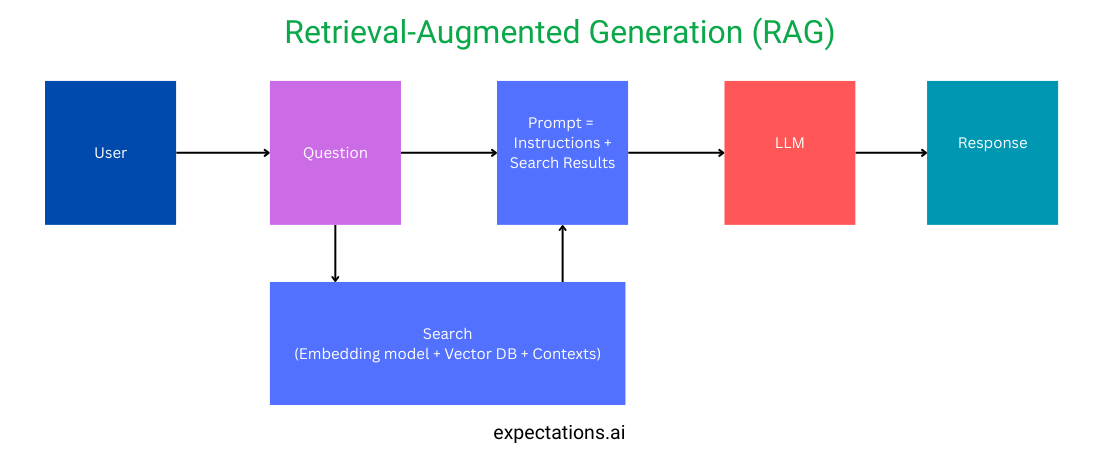
How It Fits in the IBM Watsonx Suite
Component | Role |
---|---|
watsonx.ai | Train, tune, and deploy foundation models |
watsonx.data | Manage and query data for AI workloads |
watsonx.governance | Govern AI use with policy, tracking, explainability |
Together, they offer end-to-end AI lifecycle management — from data to model to deployment to compliance.